Cytometry Single-Cell Imaging
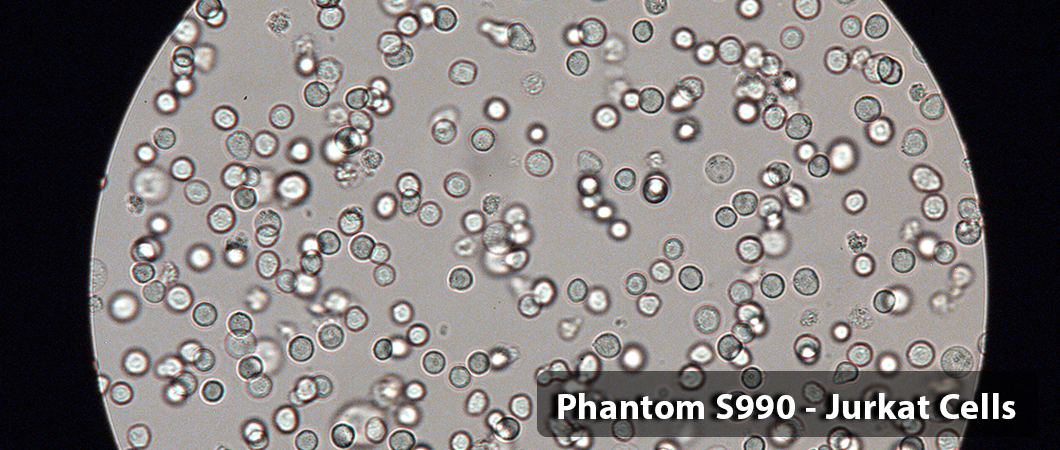
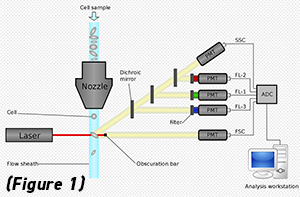
Flow Cytometry
Flow cytometry is a well-established method often used by biologists and biomedical engineers to characterize individual cells that are suspended in solution. In conventional flow cytometry, these cells pass through a microchannel and are probed with a laser. The laser-cell interaction causes either photon-scattering (to get granularity and size) and/or photo-absorption and fluorescence emission (to detect molecular markers on the cells). Finally, data processing software takes the scattering and fluorescence signals and combines them such that important statistics can be garnered from the given population of cells. This technique has even been extended to cell sorting, where a heterogeneous mixture of cells can be rapidly sorted based on the individual scattering and fluorescence signal of each cell.
(Figure 1: Illustration of flow cytometry. Image from Wiki.)
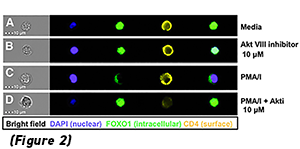
Image Cytometry
Image cytometry is similar to flow cytometry, but where images of the cells are acquired as they flow through a microchannel. This is done with two primary imaging modes: bright-field and fluorescence imaging. From a bright-field image, the physical characteristics of cells can be discerned — size, shape, opacity, granularity, elasticity, and so on. On the other hand, a fluorescence image is used to identify both the type and distribution of key biomarkers present in each cell. (Figure 2: Illustration of prototypical data from image cytometry. Image from GEN )